About bNoteable
bNoteable helps you showcase your hard work on a path to reach your goals by leveraging your band, orchestra, or vocal experience to its fullest potential to college admissions boards.
This begins early by setting a course that allows you to turn those hours of fun and friendship into leadership experience, hours of practice and performances into scholarship potential, and years of music classes into overall higher SATs and GPA scores, and academic achievement.
Executive Summary
Continuing the development of a musician networking platform which involved implementing new features, enhancing the existing ones, and fixing bugs/errors/issues in the platform by improving its efficiency and productivity along with making the platform responsive.
Problem Statement
Our client wanted us to design and create a social platform where each and every user is able to connect and interact with one another easily. He came to us after a bad experience with some other company and was expecting to continue the development by improving website performance as well as efficiency.
The platform had various bugs which needed to be fixed and some major features were to be added like payment service, OTP service, adding more security along with improving existing features. Performance of platform was being affected as there were some major issues like:
1. Deployment architecture- Everything was deployed on a single EC2 instance due to which there was a high amount of downtime. The performance was impacted more when the user base was increased.
2. The videos on his platform were taking a lot of time to load.
Our Solutions
1) We followed MVC architecture for developing REST API using express as middleware and mongoose for managing data in MongoDB. Authenticated API with jwt by using JSON web token package.
2) Added payment service in the platform by integrating stripe payment gateway with help of stripe package, created OTPs for security/validation which was communicated via SMS with help of Twilio.
3) To improve the performance, we deployed the backend on a separate ec2 instance with Nginx as reverse proxy and pm2 as process manager which comes with a built-in load balancer and helps to keep the application alive forever.
4) Installed Nginx on the server, and changed the Nginx.conf file configurations as per the requirement and it worked as a load balancing solution. Also replaced the lets encrypt SSL certificates with ACM(AWS Certificate Manager) to make certificate renewal, provision, and management process better as well as easy.
5) For adding new features to the platform, the frontend involved creating several components, services, directives, pipes, and modules in Angular.
6) To reduce the load time we implemented Lazy loading with help of Lazy load routes. The reason behind increased load time for videos was the use of video tag over secured protocol, to solve this we used iframe for rendering videos which proved to be much faster.
7) Changed the existing deployment architecture and moved the front-end to S3 so that load on the server can be reduced. We moved the front-end to S3 with CloudFront as CDN for speeding up the distribution of web content and improving performance.
Technologies
Angular 10, Node, Express, MongoDB, AWS S3, EC2, CloudFront
Success Metrics
1. Provided all the deliverables within the expected deadlines, improved performance as down time reduced and videos were no longer buffering for a long time.
2. Met all the expectations of the client and with positive feedback. All his meetings with directors and students were successful due to which he wanted us to implement some more new features on his platform.
3. Continuous reporting of progress to the client.
‚Äç
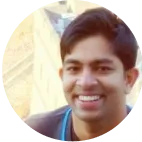
Cloudtech Achieves the AWS Service Delivery Designation for AWS Lambda
Related Resources
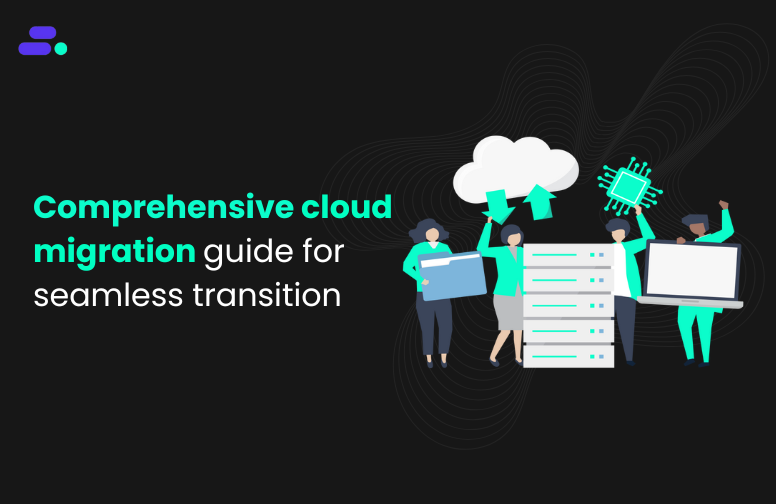
Cloud migration has become an essential process for businesses seeking to improve efficiency, reduce costs, and scale operations. For small and medium-sized businesses (SMBs), transitioning to the cloud offers the opportunity to move away from traditional IT infrastructures, providing access to flexible resources, enhanced security, and the ability to innovate more quickly.
One study shows the global cloud migration services market was valued at approximately $10.91 billion in 2023 and is projected to grow to $69.73 billion by 2032, at a CAGR of 23.9%. This growth reflects the increasing demand for cloud solutions across industries, making migration an imperative step for businesses looking to stay competitive.
However, migrating to the cloud isn't as simple as just shifting data—there are key steps to ensure a smooth transition. This guide will walk businesses through the entire process, from initial planning to execution, helping them avoid common pitfalls and achieve the best outcomes for their cloud migration.
What is cloud migration?
Cloud migration is the method of moving a company's data, business elements, and other applications from on-premises infrastructure to cloud-based systems. This transition allows businesses to access scalable resources, reduce operational costs, and improve flexibility by using the cloud’s storage, computing, and network capabilities.
Cloud migration can involve moving entirely to the cloud or using a hybrid model, where some data and applications remain on-site while others are hosted in the cloud. The process typically includes planning, data transfer, testing, and ensuring everything works smoothly in the new cloud environment. It is a crucial step for businesses looking to modernize their IT infrastructure.
What are the benefits of cloud migration?
Cloud migration allows SMBs to improve efficiency and reduce costs by moving away from traditional IT infrastructure.
- Lower IT costs: Traditional IT infrastructure can be expensive to maintain, with costs for hardware, software, and support adding up quickly. Cloud migration helps businesses cut these costs by eliminating the need for expensive on-site equipment and offering a pay-as-you-go model. This makes it easier for businesses to manage budgets and save money.
- Flexibility to scale: Many small businesses face challenges when their needs grow, leading to expensive IT upgrades. The cloud offers the flexibility to easily scale resources up or down so companies can adjust to fluctuating requirements without the financial burden of over-investing in infrastructure.
- Enhanced security without extra effort: Data breaches and security concerns can be a major headache for small businesses that may not have the resources to manage complex security systems. Cloud providers offer top-tier security features, like encryption and regular audits, giving businesses peace of mind while saving them time and effort on security management.
- Remote access and collaboration: With more teams working remotely, staying connected can be a challenge. Cloud migration allows employees to access files and collaborate from anywhere, making it easier to work across locations and teams without relying on outdated, on-premises systems.
- Reliable backup and disaster recovery: Losing important business data can be devastating, especially for smaller companies that can't afford lengthy downtime. Cloud migration solutions may include disaster recovery features, which help automatically back up data, reducing the risk of data loss and allowing for quicker recovery in case of unforeseen issues.
- Automatic updates, less maintenance: Small businesses often struggle to keep their systems up to date, leading to security vulnerabilities or performance issues. Cloud migration ensures that the provider handles software updates and maintenance automatically, so businesses can focus on what they do best instead of worrying about IT.
7 R's cloud migration strategies for SMBs to consider
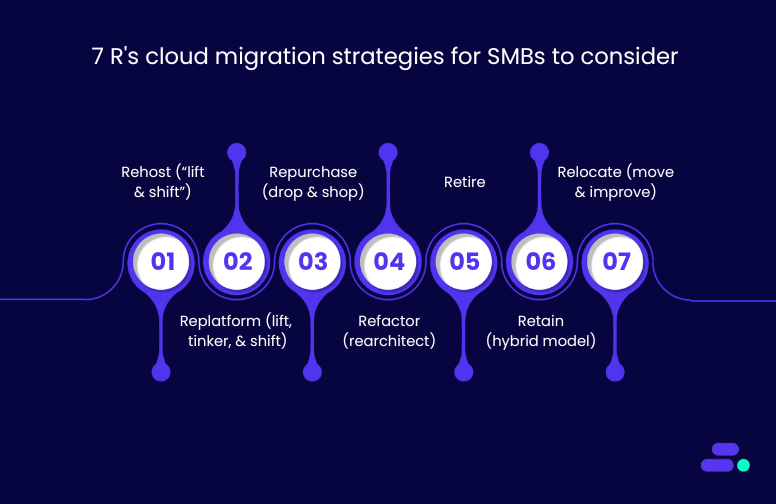
The concept of the 7 R’s of cloud migration emerged as organizations began facing the complex challenge of moving diverse applications and workloads to the cloud. As early adopters of cloud technology quickly discovered, there was no one-size-fits-all approach to migration. Each system had different technical requirements, business priorities, and levels of cloud readiness. To address this, cloud providers and consulting firms began categorizing migration strategies into a structured framework.
Each "R" represents a strategy for efficiently migrating companies' infrastructure to the cloud. Here’s a breakdown of each strategy:
- Rehost (lift and shift): Rehost (lift and shift) involves moving applications to the cloud with minimal changes, offering a fast migration but not utilizing cloud-native features like auto-scaling or cost optimization.
This is the simplest and quickest cloud migration strategy. It entails transferring applications and data to the cloud with few adjustments, essentially “lifting” them from on-premises servers and “shifting” them to the cloud. While this method requires little modification, it may not take full advantage of cloud-native features like scalability and cost savings.
When to use: Ideal for businesses looking for a fast migration, without altering existing applications significantly.
- Replatform (lift, tinker, and shift): Replatforming involves making minor adjustments to applications before migrating them to the cloud. This could mean moving to a different database service or tweaking configurations for cloud compatibility. Replatforming ensures applications run more efficiently in the cloud without a complete redesign.
When to use: Suitable for businesses wanting to gain some cloud benefits like improved performance or cost savings, without a complete overhaul of their infrastructure.
- Repurchase (drop and shop): This strategy involves replacing an existing application with a cloud-native solution, often through Software-as-a-Service (SaaS) offerings. For instance, a business might move from an on-premises CRM to a cloud-based CRM service. Repurchasing is often the best choice for outdated applications that are no longer cost-effective or efficient to maintain.
When to use: Best when an organization wants to adopt modern, scalable cloud services and replace legacy systems that are costly to maintain.
- Refactor (rearchitect): Refactoring, or rearchitecting, involves redesigning an application to leverage cloud-native features fully. This may include breaking down a monolithic application into microservices or rewriting parts of the codebase to improve scalability, performance, or cost efficiency. Refactoring enables businesses to unlock the full potential of the cloud.
When to use: This solution is ideal for businesses with long-term cloud strategies that are ready to make significant investments to improve application performance and scalability.
- Retire: The retire strategy is about eliminating applications or workloads that are no longer useful or relevant. This might involve decommissioning outdated applications or workloads that are redundant, no longer in use, or replaced by more efficient solutions in the cloud.
When to use: When certain applications no longer serve the business and moving them to the cloud would not provide any value. - Retain (hybrid model): Retaining involves keeping some applications and workloads on-premises while others are migrated to the cloud. This is often part of a hybrid cloud strategy, where certain critical workloads remain on-site for security, compliance, or performance reasons while less critical systems move to the cloud.
When to use: This is useful for businesses with specific compliance or performance requirements that necessitate keeping certain workloads on-premises. - Relocate (move and improve): Relocate involves moving applications and workloads to the cloud, but with some minor modifications to enhance cloud performance. This strategy is a middle ground between rehosting and more extensive restructuring, allowing businesses to improve certain elements of their infrastructure to better utilize cloud features without fully re-architecting applications.
When to use: Best for companies looking to move quickly to the cloud but with some minor adjustments to take advantage of cloud features like better resource allocation.
By understanding these 7 R’s and aligning them with business goals, companies can select the most appropriate strategy for each workload, ensuring a smooth, efficient, and cost-effective cloud migration.
Phases of the cloud migration process
Cloud migration is a strategic process that helps businesses shift their data, applications, and IT infrastructure from on-premise systems to cloud-based platforms. It involves several phases, each with its own set of activities and considerations. Here's a breakdown of the key phases involved in cloud migration:
1. Assess Phase
This is the initial phase of cloud migration where the organization evaluates its current IT environment, goals, and readiness for the cloud transition. The objective is to understand the landscape before making any migration decisions.
Key activities in the Assess Phase:
- Cloud Readiness Assessment: This includes evaluating the organization’s current IT infrastructure, security posture, and compatibility with cloud environments. A detailed assessment helps in understanding if the existing systems can move to the cloud or require re-architecting.
- Workload Assessment: Companies need to assess which workloads (applications, databases, services) are suitable for migration and how they should be prioritized. This process may also involve identifying dependencies between workloads that should be considered in the migration plan.
- Cost and Benefit Analysis: A detailed cost-benefit analysis should be carried out to estimate the financial implications of cloud migration, including direct and indirect costs, such as licensing, cloud service fees, and potential productivity improvements.
At the end of the Assess Phase, the organization should have a clear understanding of which systems to migrate, a roadmap, and the necessary cloud architecture to proceed with.
2. Mobilize Phase
The Mobilize Phase is where the groundwork for the migration is laid. In this phase, the organization prepares to move from assessment to action by building the necessary foundation for the cloud journey.
Key activities in the Mobilize Phase:
- Cloud Strategy and Governance: This step focuses on defining the cloud strategy, including governance structures, security policies, compliance requirements, and budget allocation. The organization should also identify the stakeholders and roles involved in the migration process.
- Resource Planning and Cloud Setup: The IT team prepares the infrastructure on the cloud platform, including setting up virtual machines, storage accounts, databases, and networking components. Key security and monitoring tools should also be put in place to manage and track the cloud environment effectively.
- Change Management Plan: It's crucial to manage how the transition will impact people and processes. Creating a change management plan ensures that employees are informed, trained, and supported throughout the migration process.
By the end of the Mobilize Phase, the organization should be fully prepared for the actual migration process, with infrastructure set up and a clear plan in place to manage the change.
3. Migrate and Modernize Phase
The Migrate and Modernize Phase is the heart of the migration process. This phase involves actual migration, along with the modernization of legacy applications and IT systems to take full advantage of the cloud.
Migration Stage 1: Initialize
In the Initialize stage, the organization starts by migrating the first batch of applications or workloads to the cloud. This stage involves:
- Defining Migration Strategy: Organizations decide on a migration approach—whether it’s rehosting (lift and shift), replatforming (moving to a new platform with some changes), or refactoring (re-architecting applications for the cloud).
- Pilot Testing: Before fully migrating all workloads, a pilot migration is performed. This allows teams to test and validate cloud configurations, assess the migration process, and make any necessary adjustments.
- Addressing Security and Compliance: Ensuring that security and compliance policies are in place for the migrated applications is key. During this phase, security tools and practices, like encryption and access control, are configured for cloud environments.
The Initialize stage essentially sets the foundation for a successful migration by moving a few workloads and gathering lessons learned to adjust the migration strategy.
Migration Stage 2: Implement
The Implement stage is the execution phase where the full-scale migration occurs. This stage involves:
- Full Migration Execution: Based on the lessons from the Initialize stage, the organization migrates all identified workloads, databases, and services to the cloud.
- Modernization: This is the phase where the organization takes the opportunity to modernize its legacy systems. This might involve refactoring applications to take advantage of cloud-native features, such as containerization or microservices architecture, improving performance, scalability, and cost-efficiency.
Integration and Testing: Applications and data are fully integrated with the cloud environment. Testing ensures that all systems are working as expected, including testing for performance, security, and functionality. - Performance Optimization: Once everything is in place, performance optimization becomes a priority. This may involve adjusting resources, tuning applications for the cloud, and setting up automation for scaling based on demand.
At the end of the Implement stage, the migration is considered complete, and the organization should be fully transitioned to the cloud with all systems functional and optimized for performance.
Common cloud migration challenges
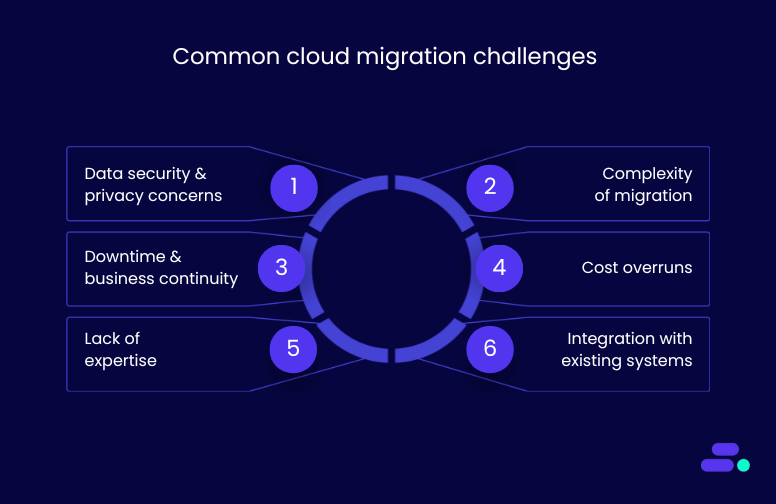
While cloud migration offers numerous benefits, it also comes with its own set of challenges. Understanding these hurdles can help SMBs prepare and ensure a smoother transition.
- Data security and privacy concerns: Moving sensitive data to the cloud can raise concerns about its security and compliance with privacy regulations. Many businesses worry about unauthorized access or data breaches. Ensuring that the cloud provider offers strong security protocols and compliance certifications is crucial to addressing these fears.
- Complexity of migration: Migrating data, applications, and services to the cloud can be a tricky procedure, especially for businesses with legacy systems or highly customized infrastructure. The challenge lies in planning and executing the migration without causing significant disruptions to ongoing operations. It requires thorough testing, proper tool selection, and a well-defined migration strategy.
- Downtime and business continuity: Businesses fear downtime during the migration process, as it could impact productivity, customer experience, and revenue. Planning for minimal downtime with proper testing, backup solutions, and scheduling during off-peak hours is vital to mitigate this risk.
- Cost overruns: While cloud migration is often seen as a cost-saving move, without proper planning, businesses may experience unexpected costs. This could be due to hidden fees, overspending on resources, or underestimating the complexity of migrating certain workloads. It’s essential to budget carefully and select the right cloud services that align with the business’s needs.
- Lack of expertise: Many small businesses lack the in-house expertise to execute a cloud migration effectively. Without knowledgeable IT staff, businesses may struggle to manage the migration process, leading to delays, errors, or suboptimal cloud configurations. In such cases, seeking external help from experienced cloud consultants can alleviate these concerns.
- Integration with existing systems: One of the biggest challenges is ensuring that cloud-based systems integrate smoothly with existing on-premises infrastructure and other third-party tools. Poor integration can lead to inefficiencies and system incompatibilities, disrupting business operations.
If you are already migrated to the cloud, partners like Cloudtech help SMBs modernize their cloud environments for better performance, scalability, and cost-efficiency. Unlock the full potential of your existing cloud infrastructure with expert optimization and support from Cloudtech. Get in touch to future-proof your cloud strategy today.
Conclusion
In conclusion, cloud migration offers small and medium-sized businesses significant opportunities to improve efficiency, scalability, and cost-effectiveness. By following the right strategies and best practices, businesses can achieve a seamless transition to the cloud while addressing common challenges.
For businesses looking to optimize their cloud services, Cloudtech provides tailored solutions to streamline the process, from infrastructure optimization to application modernization. Use Cloudtech’s expertise to unlock the full potential of cloud technology and support your business growth.
Frequently Asked Questions (FAQs)
1. What is cloud migration, and why is it important?
A: Cloud migration is the process of moving digital assets, such as data, applications, and IT resources, from on-premises infrastructure to cloud environments. It is important because it enables businesses to improve scalability, reduce operational costs, and increase agility in responding to market demands.
2. What are the 7 R’s of cloud migration, and how do they help?
A: The 7 R’s include Rehost, Replatform, Refactor, Repurchase, Retire, Retain, and Relocate. It represents strategic approaches businesses can use when transitioning workloads to the cloud. This framework helps organizations evaluate each application individually and choose the most effective migration method based on technical complexity, cost, and business value.
3. How can a small business prepare for a successful cloud migration?
A: Small businesses should start by assessing their current IT environment, setting clear goals, and identifying which workloads to move first. It's also crucial to allocate a realistic budget, ensure data security measures are in place, and seek external support if internal expertise is limited.
4. What challenges do SMBs commonly face during cloud migration?
A: SMBs often face challenges such as limited technical expertise, data security concerns, cost overruns, and integration issues with legacy systems. Many struggle with creating a well-structured migration plan, which can lead to downtime and inefficiencies if not properly managed.
5. How long does a typical cloud migration take?
A: The duration of a cloud migration depends on the size and complexity of the infrastructure being moved. It can range from a few weeks for smaller, straightforward migrations to several months for large-scale or highly customized environments. Proper planning and execution are key to minimizing delays.
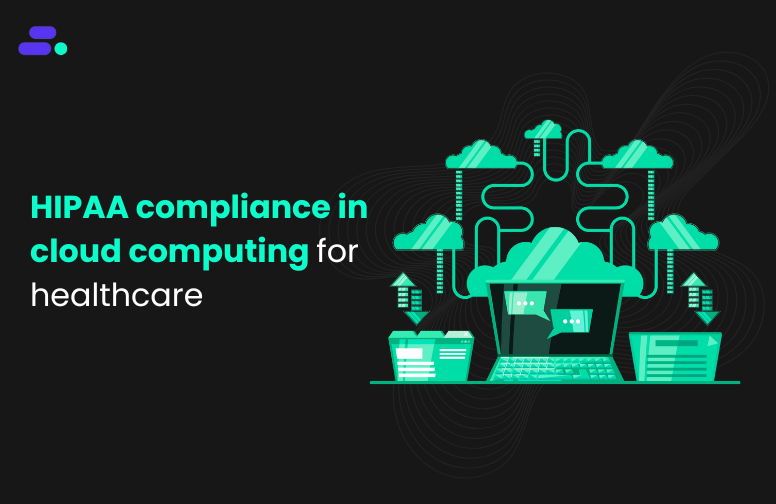
Small and mid-sized businesses (SMBs) in the healthcare sector are increasingly turning to cloud solutions to streamline operations, improve patient care, and reduce infrastructure costs. In fact, a recent study revealed that 70% of healthcare organizations have adopted cloud computing solutions, with another 20% planning to migrate within the next two years, indicating a 90% adoption rate by the end of 2025.
However, with the shift to digital platforms comes the critical responsibility of maintaining compliance with the Health Insurance Portability and Accountability Act (HIPAA). It involves selecting cloud providers that meet HIPAA requirements and implementing the right safeguards to protect sensitive patient data.
In this blog, we will look at how healthcare SMBs can stay HIPAA-compliant in the cloud, address their specific challenges, and explore how cloud solutions can help ensure both security and scalability for their systems.
Why HIPAA compliance is essential for cloud computing in healthcare
With the rise of cloud adoption, healthcare SMBs must ensure they meet HIPAA standards to protect data and avoid legal complications. Here are three key reasons why HIPAA compliance is so important in cloud computing for healthcare:
- Safeguarding electronic Protected Health Information (ePHI): HIPAA regulations require healthcare organizations to protect sensitive patient data, ensuring confidentiality and security. Cloud providers offering HIPAA-compliant services implement strong encryption methods and other security measures to prevent unauthorized access to ePHI.
- Mitigating risks of data breaches: Healthcare organizations are prime targets for cyberattacks, and data breaches can result in significant financial penalties and loss of trust. HIPAA-compliant cloud solutions provide advanced security features such as multi-factor authentication, secure data storage, and regular audits to mitigate these risks and prevent unauthorized access to patient data.
- Ensuring privacy and security of patient data: HIPAA ensures overall privacy and security beyond just ePHI protection. Cloud environments that comply with HIPAA standards implement safeguards that protect patient data both at rest and in transit, ensuring that healthcare organizations meet privacy requirements and provide patients with the peace of mind they deserve.
By maintaining HIPAA compliance in the cloud, healthcare organizations can also build trust with patients, safeguard valuable data, and streamline their operations.
Benefits of cloud computing for healthcare
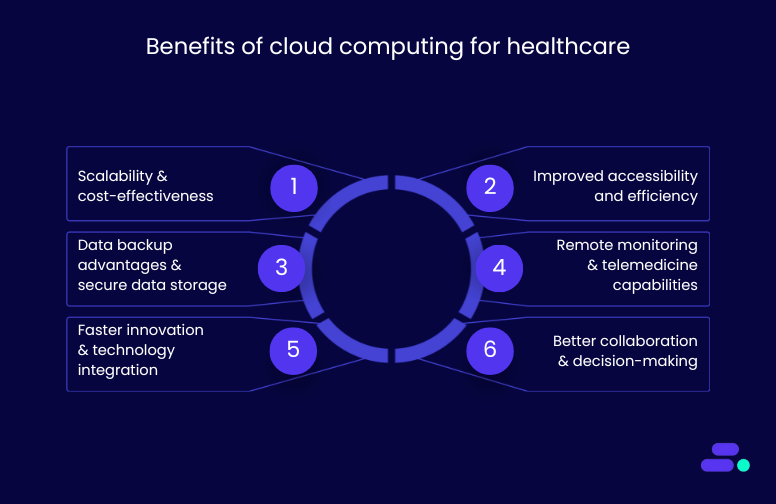
Cloud computing is reshaping the healthcare landscape, providing significant advantages that enhance service delivery, operational efficiency, and patient care. Here are some key benefits healthcare organizations can experience by adopting cloud solutions:
- Scalability and cost-effectiveness: Cloud computing allows healthcare organizations to adjust their infrastructure as needed, reducing the need for expensive hardware investments and offering pay-as-you-go models, making it ideal for SMBs with fluctuating demands.
- Improved accessibility and efficiency: Cloud-based systems enable healthcare teams to securely access, streamlining communication and speeding up diagnosis and treatment decisions. Administrative tasks also become more efficient, allowing healthcare professionals to focus on patient care.
- Reliable data backup and secure storage: Cloud computing provides backup solutions that ensure patient data is securely stored and easily recoverable in case of system failure or disaster, ensuring minimal downtime and business continuity.
- Remote monitoring and telemedicine capabilities: Cloud platforms facilitate remote patient monitoring and telemedicine, allowing healthcare providers to offer care to patients in underserved or remote areas, thus improving access and patient outcomes.
- Faster innovation and technology integration: Cloud infrastructure enables healthcare organizations to quickly adopt new technologies like artificial intelligence (AI) and machine learning (ML), enhancing decision-making and enabling personalized care by efficiently analyzing large patient data sets.
Cloud-native innovations such as serverless computing and container orchestration (e.g., AWS Lambda and Amazon EKS) enable SMBs to improve compliance and scalability simultaneously, reducing operational complexity and risk. - Better collaboration and Decision-making: With cloud computing, real-time data sharing improves collaboration among healthcare teams across locations, ensuring decisions are based on the most current information and fostering more effective teamwork.
By using cloud computing, healthcare providers can improve their operational efficiency, reduce costs, and offer better, more accessible care to their patients.
HIPAA compliance requirements in cloud computing
Cloud computing is transforming healthcare by improving service quality, boosting operational efficiency, and enabling better patient outcomes. Below are the main HIPAA compliance factors to focus on:
1. Business associate agreements (BAAs) with cloud service providers (CSPs)
A Business Associate Agreement (BAA) is a legally binding contract between healthcare organizations and their cloud service providers (CSPs). The BAA outlines the provider’s responsibility to protect PHI (Protected Health Information) and comply with HIPAA regulations. Without a signed BAA, healthcare organizations cannot ensure that their CSP is following the necessary security and privacy protocols.
2. Ensuring data encryption at rest and in transit
To maintain HIPAA compliance, healthcare SMBs must ensure that Protected Health Information (PHI) is encrypted both at rest (when stored on cloud servers) and in transit (during transmission).
- Data at rest: PHI must be encrypted when stored on cloud servers to prevent unauthorized access in case of a breach.
- Data in transit: Encryption is also required when PHI is transmitted between devices and the cloud to protect against data interception during transit.
Encryption standards such as AES-256 are commonly used to meet HIPAA’s stringent data protection requirements.
3. Implementation of access controls and audit logging
To ensure HIPAA compliance, healthcare SMBs must implement access controls that limit PHI access to authorized personnel based on their roles (RBAC).
- Access controls: Only authorized personnel should have access to PHI. Role-based access control (RBAC) helps ensure that employees can only access the data necessary for their specific role.
- Audit logging: Cloud systems must include comprehensive audit logs that track all access to PHI, documenting who accessed data, when, and why. These logs are crucial for security audits and identifying unauthorized access.
4. Regular security risk assessments
Healthcare SMBs should perform regular security risk assessments to identify vulnerabilities in their cloud infrastructure.
- Evaluate cloud providers' security practices: Conduct penetration testing and ensure an effective disaster recovery plan to help mitigate threats and maintain HIPAA compliance.
- Ensure an efficient disaster recovery plan: The risk assessments include evaluating the cloud service provider’s security practices, conducting penetration testing, and ensuring their disaster recovery plan is efficient.
By regularly assessing security, organizations can mitigate potential threats and maintain HIPAA compliance.
5. Data backup and disaster recovery
Cloud providers must offer reliable data backup and disaster recovery options to protect patient data from loss. Healthcare organizations should ensure that backup solutions meet HIPAA standards, such as geographically dispersed storage for redundancy and quick data recovery. In case of a system failure or breach, quick recovery is essential to minimize downtime and maintain service continuity.
6. Vendor management and third-party audits
Healthcare organizations must ensure that their cloud service providers and any third-party vendors follow HIPAA guidelines. Regular third-party audits should be conducted to verify that CSPs comply with HIPAA security and privacy standards. Organizations should work with their CSPs to address audit findings promptly and implement necessary improvements.
Addressing these areas helps mitigate risks associated with cloud adoption, enabling healthcare organizations to meet regulatory standards and continue delivering high-quality care.
Also Read: Building HIPAA-compliant applications on the AWS cloud.
To meet these compliance requirements, healthcare SMBs need to implement proactive strategies that protect patient data and align with HIPAA regulations.
Strategies for maintaining HIPAA compliance in the cloud
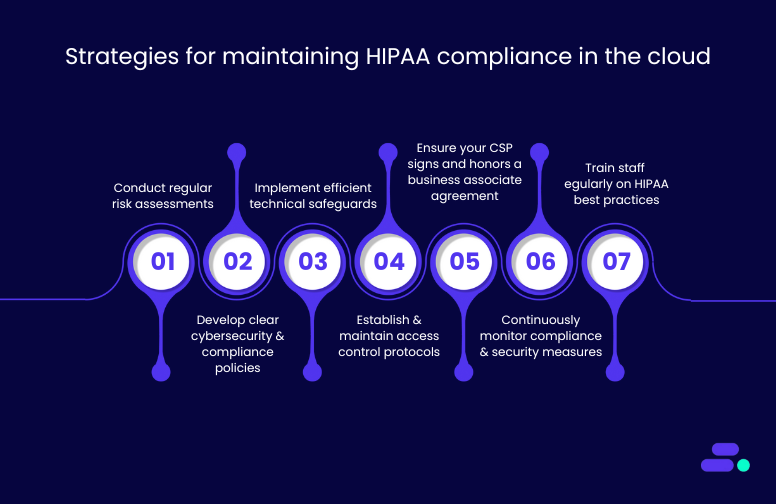
Healthcare organizations—especially SMBs—must adopt proactive and structured strategies to meet HIPAA requirements while leveraging the benefits of cloud computing. These strategies help protect sensitive patient data and maintain regulatory alignment across cloud environments.
- Conduct regular risk assessments: Identify vulnerabilities across all digital systems, including cloud platforms. Evaluate how electronic Protected Health Information (ePHI) is stored, accessed, and transmitted. Use risk assessment insights to strengthen internal policies and address compliance gaps.
- Develop clear cybersecurity and compliance policies: Outline roles, responsibilities, and response plans in the event of a breach. Policies should align with HIPAA rules and be regularly updated to reflect evolving cloud practices and threat landscapes.
- Implement efficient technical safeguards: Use firewalls, intrusion detection systems, and end-to-end encryption to secure data both at rest and in transit. Ensure automatic data backups and redundancy systems are in place for data recovery.
Adopting Infrastructure as Code (IaC) tools like Terraform or AWS CloudFormation allows SMBs to automate security policy enforcement and maintain consistent, auditable configurations aligned with HIPAA requirements. - Establish and maintain access control protocols: Adopt role-based access, strong password requirements, and multi-factor authentication. Limit ePHI access to only those who need it and track access through detailed audit logs.
- Ensure CSP signs and complies with a business associate agreement (BAA): This agreement legally binds the cloud provider to uphold HIPAA security standards. It’s a non-negotiable element to use any third-party service to handle ePHI.
- Continuously monitor compliance and security measures: Regularly review system activity logs and CSP practices to confirm adherence to HIPAA standards. Leverage cloud-native monitoring tools for real-time alerts and policy enforcement.
- Train staff regularly on HIPAA best practices: Human error remains a leading cause of data breaches. Conduct frequent training sessions to keep teams informed on compliance policies, security hygiene, and breach response procedures.
By integrating these strategies, healthcare SMBs can confidently move forward in their cloud adoption journey while upholding the trust and safety of their patient data.
Choosing a HIPAA-compliant cloud service provider
Selecting the right cloud service provider (CSP) is critical for healthcare organizations looking to maintain HIPAA compliance. A compliant CSP should not only offer secure infrastructure but also demonstrate a clear understanding of HIPAA’s specific requirements for ePHI.
- Evaluate the CSP’s compliance certifications and track record: Look for providers that offer documented proof of compliance, such as HITRUST CSF, ISO/IEC 27001, or SOC 2 Type II. A strong compliance posture indicates the provider is prepared to handle sensitive healthcare data responsibly.
- Verify their willingness to sign a Business Associate Agreement (BAA): Under HIPAA, any third-party that handles ePHI is considered a business associate. A CSP must agree to sign a BAA, legally committing to uphold HIPAA security and privacy requirements. Without this agreement, working with the provider is non-compliant.
- Assess security features tailored for healthcare data: Choose CSPs that provide built-in encryption (at rest and in transit), detailed audit logging, role-based access controls, and real-time monitoring. These tools help healthcare SMBs meet HIPAA’s technical safeguard requirements.
- Review the provider’s shared responsibility model: Understand which aspects of security and compliance are managed by the CSP and which are the responsibility of the customer. A transparent shared responsibility model avoids compliance gaps and misconfigurations.
- Evaluate support and incident response capabilities: Choose a provider that offers 24/7 technical support, a clear escalation path for security incidents, and defined recovery time objectives. A timely response can minimize the impact of breaches or service disruptions.
- Consider the CSP’s experience in healthcare: A provider familiar with healthcare clients will be better equipped to meet HIPAA expectations. Look for case studies or client references that demonstrate success in the healthcare space.
By thoroughly vetting potential cloud providers through these criteria, healthcare organizations can make informed decisions that reduce risk and ensure compliance from the ground up.
Cloudtech helps your business achieve and maintain HIPAA compliance in the cloud, without compromising on performance or scalability. With Cloudtech, you get expert guidance, ongoing compliance support, and a secure infrastructure built to handle sensitive patient data.
Challenges and risks of cloud computing in healthcare
While cloud computing offers numerous benefits, it also presents specific challenges that healthcare organizations must address to stay compliant and secure.
- Management of shared infrastructure and potential compliance issues: Cloud environments often operate on a shared infrastructure model, where multiple clients access common resources. Without strict isolation and proper configuration, this shared model can increase the risk of unauthorized access or compliance violations.
- Handling security and privacy concerns effectively: Healthcare data is a prime target for cyberattacks. Ensuring encryption, access controls, and real-time monitoring is essential. However, gaps in internal policies or misconfigurations can lead to breaches, even with advanced cloud tools in place.
- Dealing with jurisdictional issues related to cloud data storage: When cloud providers store data across multiple geographic locations, regulatory conflicts may arise. Data residency laws vary by country and can impact how patient information is stored, accessed, and transferred. Healthcare organizations must ensure their provider aligns with regional legal requirements.
- Maintaining visibility and control over cloud resources: As services scale, it can become difficult for internal teams to maintain oversight of all assets, configurations, and user activity. Without proper governance, this lack of visibility can increase the risk of non-compliance and delayed incident response.
- Ensuring staff training and cloud literacy: Adopting cloud technology requires continuous training for IT and administrative staff. Misuse or misunderstanding of cloud tools can compromise security or lead to HIPAA violations, even with strong technical safeguards in place.
To overcome these challenges, healthcare organizations should follow best practices to ensure continuous HIPAA compliance and safeguard patient data.
Best practices for ensuring HIPAA compliance
Healthcare organizations using the cloud must follow proven practices to protect patient data and stay HIPAA compliant.
- Sign business associate agreements (BAAs): Ensure the cloud service provider signs a BAA, clearly defining responsibilities for handling ePHI and meeting HIPAA standards.
- Enforce access controls and monitor activity: Restrict access based on roles and monitor data activity through audit logs and alerts to catch and address unusual behavior early.
- Respond quickly to security incidents: Have a clear incident response plan to detect, contain, and report breaches promptly, following HIPAA’s Breach Notification Rule.
- Conduct regular risk assessments: Periodic reviews of the cloud setup help spot vulnerabilities and update safeguards to meet current HIPAA requirements.
- Train staff on HIPAA and cloud security: Educate employees on secure data handling and how to avoid common threats like phishing to reduce human error.
Conclusion
As healthcare organizations, particularly SMBs, move forward with digital transformation, ensuring HIPAA compliance in cloud computing is both a necessity and a strategic advantage. Protecting electronic protected health information (ePHI), reducing the risk of data breaches, and benefiting from scalable, cost-effective solutions are key advantages of HIPAA-compliant cloud services.
However, achieving compliance is not just about using the right technology; it requires a comprehensive strategy, the right partnerships, and continuous monitoring.
Looking for a reliable partner in HIPAA-compliant cloud solutions?
Cloudtech provides secure, scalable cloud infrastructure designed to meet HIPAA standards. With a focus on encryption and 24/7 support, Cloudtech helps organizations protect patient data while embracing the benefits of cloud technology.
FAQs
- What is HIPAA compliance in cloud computing?
HIPAA compliance in cloud computing ensures that cloud service providers (CSPs) and healthcare organizations adhere to strict regulations for protecting patient data, including electronic Protected Health Information (ePHI). This includes data encryption, secure storage, and ensuring privacy and security throughout the data lifecycle.
- How can healthcare organizations ensure their cloud service provider is HIPAA-compliant?
Healthcare organizations should ensure their cloud service provider signs a Business Associate Agreement (BAA), provides encryption methods (both at rest and in transit), and offers secure access controls, audit logging, and real-time monitoring to protect ePHI.
- What are the key benefits of using cloud computing for healthcare organizations?
Cloud computing provides healthcare organizations with scalability, improved accessibility, cost-effectiveness, enhanced data backup, and disaster recovery solutions. Additionally, it supports remote monitoring and telemedicine, facilitating more accessible patient care and improved operational efficiency.
- What are the consequences of non-compliance with HIPAA regulations in cloud computing?
Non-compliance with HIPAA regulations can lead to severe penalties, including hefty fines and damage to an organization’s reputation. It can also result in unauthorized access to sensitive patient data, leading to breaches of patient privacy and trust.
- What should be included in a HIPAA-compliant cloud security strategy?
A HIPAA-compliant cloud security strategy should include regular risk assessments, encryption of ePHI, access control mechanisms, audit logging, a disaster recovery plan, and ongoing staff training. Additionally, healthcare organizations should ensure their cloud provider meets all HIPAA technical safeguards and legal obligations.
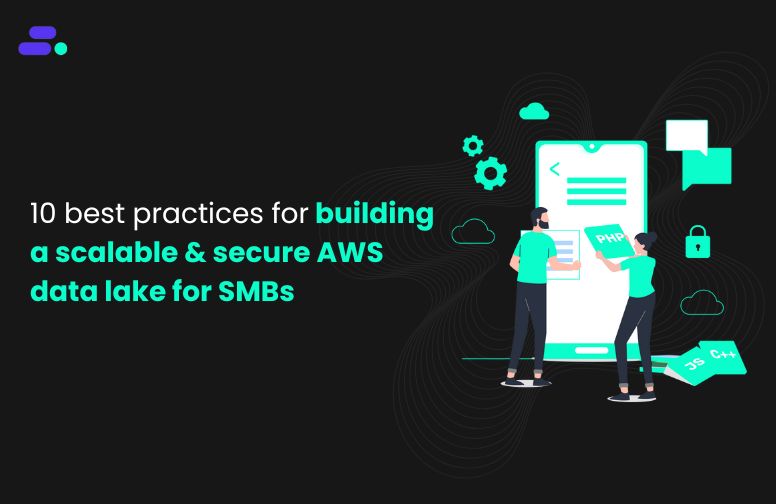
Data is the backbone of all business decisions, especially when organizations operate with tight margins and limited resources. For SMBs, having data scattered across spreadsheets, apps, and cloud folders can hinder efficiency.
According to Gartner, poor data quality costs businesses an average of $12.9 million annually. SMBs cannot afford such inefficiency. This is where an Amazon Data Lake proves invaluable.
It offers a centralized and scalable storage solution, enabling businesses to store all their structured and unstructured data in one secure and searchable location. It also simplifies data analysis. In this guide, businesses will discover 10 practical best practices to help them build an AWS data lake that aligns with their specific goals.
What is an Amazon data lake, and why is it important for SMBs?
An Amazon Data Lake is a centralized storage system built on Amazon S3, designed to hold all types of data, whether it comes from CRM systems, accounting software, IoT devices, or customer support logs. Businesses do not need to convert or structure the data beforehand, which saves time and development resources. This makes data lakes particularly suitable for SMBs that gather data from multiple sources but lack large IT teams.
Traditional databases and data warehouses are more rigid. They require pre-defining data structures and often charge based on compute power, not just storage. A data lake, on the other hand, flips that model. It gives businesses more control, scales with growth, and facilitates advanced analytics, all without the high overhead typically associated with traditional systems.
To understand how an Amazon data lake works, it helps to know the five key components that support data processing at scale:
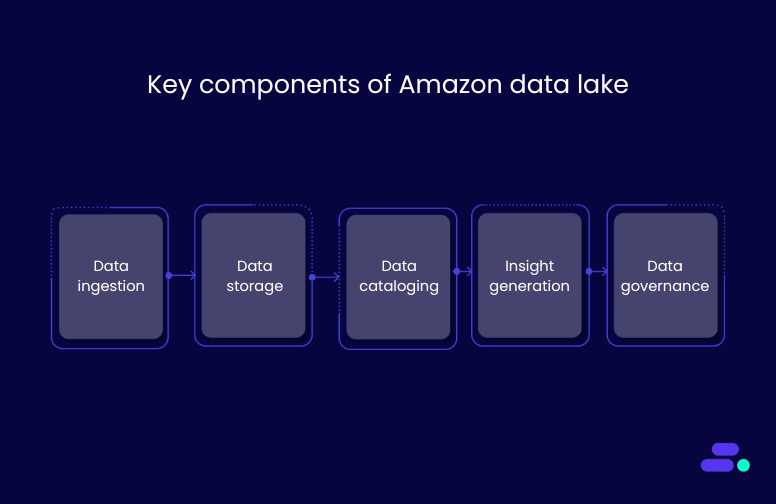
- Data ingestion: Businesses can bring in data from both cloud-based and on-premises systems using tools designed to efficiently move data into Amazon S3.
- Data storage: All data is stored in Amazon S3, a highly durable and scalable object storage service.
- Data cataloging: Services like AWS Glue automatically index and organize data, making it easier for businesses to search, filter, and prepare data for analysis.
- Data analysis and visualization: Data lakes can be connected to tools like Amazon Athena or QuickSight, enabling businesses to query, visualize, and uncover insights directly without needing to move data elsewhere.
- Data governance: Built-in controls such as access permissions, encryption, and logging help businesses manage data quality and security. Amazon S3 access logs can track user actions, and permissions can be enforced using AWS IAM roles or AWS Lake Formation.
Why an Amazon data lake matters for business
- Centralized access: Businesses can store all their data from product inventory to customer feedback in one place, accessible by teams across departments.
- Flexibility for all data types: Businesses can keep JSON files, CSV exports, videos, PDFs, and more without needing to transform them first.
- Lower costs at scale: With Amazon S3, businesses only pay for the storage they use. They can use Amazon S3 Intelligent-Tiering to reduce costs as data becomes less frequently accessed.
- Access to advanced analytics: Businesses can run SQL queries with Amazon Athena, train machine learning models with Amazon SageMaker, or build dashboards with Amazon QuickSight directly on their Amazon data lake, without moving the data.
With the rise of generative AI (GenAI), businesses can unlock even greater value from their Amazon data lake.
Amazon Bedrock enables SMBs to build and scale AI applications without managing underlying infrastructure. By integrating Bedrock with your data lake, you can use pre-trained foundation models to generate insights, automate data summarization, and drive smarter decision-making, all while maintaining control over your data security and compliance.
10 best practices to build a smart, scalable data lake on AWS
A successful Amazon data lake is more than just a storage bucket. It’s a living, evolving system that supports growth, analysis, and security at every stage. These 10 best practices will help businesses avoid costly mistakes and build a data lake that delivers real, measurable results.
1. Design a tiered storage architecture
Start by separating data into three functional zones:
- Raw zone: This is the original data, untouched and unfiltered. Think IoT sensor feeds, app logs, or CRM exports.
- Staging zone: Store cleaned or transformed versions here. It’s used by data engineers for processing and QA.
- Curated zone: Only high-quality, production-ready datasets go here, which are used by business teams for reporting and analytics.
This setup ensures data flows cleanly through the pipeline, reduces errors, and keeps teams from working on outdated or duplicate files.
2. Use open, compressed data formats
Amazon S3 supports many file types, but not all formats perform the same. For analytical workloads, use columnar formats like Parquet or ORC.
- They compress better than CSV or JSON, saving you storage costs.
- Tools like Amazon Athena, Amazon Redshift Spectrum, and AWS Glue process them much faster.
- You only scan the columns you need, which speeds up queries and reduces compute charges.
Example: Converting JSON logs to Parquet can cut query costs by more than 70% when running regular reports.
3. Apply fine-grained access controls
SMBs might have fewer users than large enterprises, but data access still needs control. Broad admin roles or shared credentials should be avoided.
- Roles and permissions should be defined with AWS IAM. Additionally, AWS Lake Formation provides advanced capabilities for data governance, allowing businesses to restrict access at the column or row level. For example, HR may have access to employee IDs but not salaries.
- When using AWS IAM roles within the context of AWS Lake Formation, it is crucial to tailor permissions carefully to restrict access, especially when column/row-level access controls are implemented.
- Enable audit trails so you can track who accessed what and when.
- Use AWS CloudTrail for continuous monitoring of access and changes, and Amazon Macie to automatically discover and classify sensitive data, helping maintain security and compliance.
This protects sensitive data, helps you stay compliant (HIPAA, GDPR, etc.), and reduces internal risk.
4. Tag data for lifecycle and access management
Tags are more than just labels; they are powerful tools for automation, organization, and cost tracking. By assigning metadata tags, businesses can:
- Automatically manage the lifecycle of data, ensuring that old data is archived or deleted at the right time.
- Apply granular access controls, ensuring that only the right teams or individuals have access to sensitive information.
- Track usage and generate reports based on team, project, or department.
- Tags can also feed into cost allocation reports, enabling granular tracking of storage and processing costs by project or department.
For SMBs with lean IT teams, tagging streamlines data management and reduces the need for constant manual intervention, helping to keep the data lake organized and cost-efficient.
5. Use Amazon S3 storage classes to control costs
Storage adds up, especially when you're keeping logs, backups, and historical data. Here's how to keep costs in check:
- Use Amazon S3 Standard for active data.
- Switch to Amazon S3 Intelligent-Tiering for unpredictable access.
- Amazon Glacier is intended for infrequent access, and Amazon Glacier Deep Archive is specifically designed for very long-term archival at a lower price point.
- Consider using Amazon S3 One Zone-IA (One Zone-Infrequent Access) for data that doesn't require multi-AZ resilience but needs to be accessed infrequently. This storage class offers potential cost savings.
- Set up Amazon S3 Lifecycle policies to automate transitioning data between Standard, Intelligent-Tiering, Glacier, and Deep Archive tiers, balancing cost and access needs efficiently.
Set up lifecycle policies that automatically move files based on age or access frequency. This approach helps businesses avoid unnecessary costs and ensures old data is properly managed without manual intervention.
6. Catalog everything with AWS Glue
A data lake without a catalog is like a warehouse without a map. Businesses may store vast amounts of data, but without proper organization, finding specific information becomes a challenge. For SMBs, quick access to trusted data is essential.
Businesses should use the AWS Glue Data Catalog to:
- Register and track all datasets stored in Amazon S3.
- Maintain schema history for evolving data structures.
- Enable SQL-based querying with Amazon Athena or SageMaker.
- Simplify governance by organizing data into searchable tables and databases.
7. Automate ingestion and processing
Manual uploads and data preparation do not scale. If businesses spend time moving files, they aren't focusing on analyzing them. Automating this step keeps the data lake up to date and the team focused on deriving insights.
Here’s how businesses can streamline data ingestion and processing:
- Trigger workflows using Amazon S3 event notifications when new files arrive.
- Use AWS Lambda to validate, clean, or transform data in real time.
- For larger workloads, businesses may want to consider AWS Glue or Amazon Kinesis for streaming or batch data processing in real-time, as Lambda has execution time limits that might not be ideal for large-scale data processing.
- Schedule recurring ETL jobs with AWS Glue for batch data processing.
- Reduce operational overhead and ensure data freshness without daily oversight.
- Utilize infrastructure as code tools like AWS CloudFormation or Terraform to automate data lake infrastructure provisioning, ensuring repeatability and easy updates.
8. Partition the data strategically
As data grows, so do the costs and time required to scan it. Partitioning helps businesses limit what queries need to touch, which improves performance and reduces costs.
To partition effectively:
- Organize data by logical keys like year/month/day, customer ID, or region
- Ensure each partition folder follows a consistent naming convention
- Query tools like Amazon Athena or Amazon Redshift Spectrum will scan only what’s needed
- For example, querying one month of data instead of an entire year saves time and computing cost
- Use AWS Glue Data Catalog partitions to optimize query performance, and address the small files problem by periodically compacting data files to speed up Amazon Athena and Redshift Spectrum queries.
9. Encrypt data at rest and in transit
Whether businesses are storing customer records or financial reports, security is non-negotiable. Encryption serves as the first line of defense, both in storage and during transit.
Protect your Amazon data lake with:
- S3 server-side encryption to secure data at rest
- HTTPS enforcement to prevent data from being exposed during transfer
- AWS Key Management Service (KMS) for managing, rotating, and auditing encryption keys
- Compliance with standards like HIPAA, SOC2, and PCI without adding heavy complexity
10. Monitor and audit the data lake
Businesses cannot fix what they cannot see. Monitoring and logging provide insights into data access, usage patterns, and potential issues before they impact teams or customers.
To keep their Amazon data lake accountable, businesses can use:
- AWS CloudTrail will log all API calls, access attempts, and bucket-level activity.
- Amazon CloudWatch is used to monitor usage patterns and performance issues and trigger alerts.
- AWS Config, which tracks AWS resource configurations and serves as a useful tool for auditing purposes.
- Dashboards and logs that help businesses prove compliance and optimize operations.
- Visibility that supports continuous improvement and risk management.
Common mistakes SMBs make (and how to avoid them)

Building a smart, scalable Amazon data lake requires more than just uploading data. SMBs often make critical mistakes that impact performance, costs, and security. Here’s what to avoid:
1. Dumping all data with no structure
Throwing data into a lake without organization is one of the quickest ways to create chaos. Without structure, your data becomes hard to navigate and prone to errors. This leads to wasted time, incorrect insights, and potential security risks.
How to avoid it:
- Implement a tiered architecture (raw, staging, curated) to keep data clean and organized.
- Use metadata tagging for easy tracking, access, and management.
- Set up partitioning strategies so you can quickly query the relevant data.
2. Ignoring cost control features
Without proper oversight, a data lake’s costs can spiral out of control. Amazon S3 storage, data transfer, and analytics services can add up quickly if businesses don’t set boundaries.
How to avoid it:
- Use Amazon S3 Intelligent-Tiering for unpredictable access patterns, and Amazon Glacier for infrequent access or archival data.
- Set up lifecycle policies to automatically archive or delete old data.
- Regularly audit storage and analytics usage to ensure costs are kept under control.
3. Lacking role-based access
Without role-based access control (RBAC), a data lake can become a security risk. Granting blanket access to all users increases the likelihood of accidental data exposure or malicious activity.
How to avoid it:
- Use AWS IAM roles to define who can access what data.
- Implement AWS Lake Formation to manage permissions at the table, column, or row level.
- Regularly audit who has access to sensitive data and ensure permissions are up to date.
4. Overcomplicating the tech stack
It’s tempting to integrate every cool tool and service, but complexity doesn’t equal value; it often leads to confusion and poor performance. For SMBs, simplicity and efficiency are key.
How to avoid it:
- Start with basic services (like Amazon S3, AWS Glue, and Athena) before adding layers.
- Keep integrations minimal, and make sure each service adds clear value to your data pipeline.
- Prioritize usability and scalability over over-engineering.
- Additionally, Amazon Redshift Spectrum could be an important service for SMBs who need SQL-based querying over Amazon S3 data, especially for larger datasets. While it's not an error, it’s a suggestion to consider.
These common mistakes are easy for businesses to fall into, but once they are understood, they are simple to avoid. By staying focused on simplicity, cost control, and security, businesses can ensure that their Amazon data lake serves their needs effectively.
Checklist for businesses ensuring the health of an Amazon data lake
Use this checklist to quickly evaluate the health of an Amazon data lake. Regularly checking these points ensures the data lake is efficient, secure, and cost-effective.
Zones created?
- Has data been organized into raw, staging, and curated zones?
- Are data types and access needs clearly defined for each zone?
Access policies in place?
- Are AWS IAM roles properly defined for users with specific access needs?
- Has AWS Lake Formation been set up for fine-grained permissions?
Data formats optimized?
- Is columnar format like Parquet or ORC being used for performance and cost efficiency?
- Have large files been compressed to reduce storage costs?
Costs tracked?
- Are Amazon S3's intelligent-tiering and Amazon Glacier being used to minimize storage expenses?
- Is there a regular review of Amazon S3 storage usage and lifecycle policies?
Query performance healthy?
- Has partitioning been implemented for faster and cheaper queries?
- Are queries running efficiently with services like Amazon Athena or Amazon Redshift Spectrum?
By using this checklist regularly, businesses will be able to keep their Amazon data lake running smoothly and cost-effectively, while ensuring security and performance remain top priorities.
Conclusion
Implementing best practices for an Amazon data lake offers clear benefits. By structuring data into organized zones, automating processes, and using cost-efficient storage, businesses gain control over their data pipeline. Encryption and fine-grained access policies ensure security and compliance, while optimized queries and cost management turn the data lake into an asset that drives growth, rather than a burden.
Cloud modernization is within reach for SMBs, and it doesn’t have to be a complex, resource-draining project. With the right guidance and tools, businesses can build a scalable and secure data lake that grows alongside their needs. Cloudtech specializes in helping SMBs modernize AWS environments through secure, scalable, and optimized data lake strategies—without requiring full platform migrations.
SMBs interested in improving their AWS data infrastructure can consult Cloudtech for tailored guidance on modernization, security, and cost optimization.
FAQs
1. How do businesses migrate existing on-premises data to their Amazon data lake?
Migrating data to an Amazon data lake can be done using tools like AWS DataSync for efficient transfer from on-premises to Amazon S3, or AWS Storage Gateway for hybrid cloud storage. For large-scale data, AWS Snowball offers a physical device for transferring large datasets when bandwidth is limited.
2. What are the best practices for data ingestion into an Amazon data lake?
To ensure seamless data ingestion, businesses can use Amazon Kinesis Data Firehose for real-time streaming, AWS Glue for ETL processing, and AWS Database Migration Service (DMS) to migrate existing databases into their data lake. These tools automate and streamline the process, ensuring that data remains up-to-date and ready for analysis.
3. How can businesses ensure data security and compliance in their data lake?
For robust security and compliance, businesses should use AWS IAM to define user permissions, AWS Lake Formation to enforce data access policies, and ensure data is encrypted with Amazon S3 server-side encryption and AWS KMS. Additionally, enabling AWS CloudTrail will allow businesses to monitor access and track changes for audit purposes, ensuring full compliance.
4. What are the cost implications of building and maintaining a data lake?
While Amazon S3 is cost-effective, managing costs requires businesses to utilize Amazon S3 Intelligent-Tiering for unpredictable access patterns and Amazon Glacier for infrequent data. Automating data transitions with lifecycle policies and managing data transfer costs, especially across regions, will help keep expenses under control.
5. How do businesses integrate machine learning and analytics with their data lake?
Integrating Amazon Athena for SQL queries, Amazon SageMaker for machine learning, and Amazon QuickSight for visual analytics will help businesses unlock the full value of their data. These AWS services enable seamless querying, model training, and data visualization directly from their Amazon data lake.
Get started on your cloud modernization journey today!
Let Cloudtech build a modern AWS infrastructure that’s right for your business.